Driving Business Innovation With AI Training Data Services
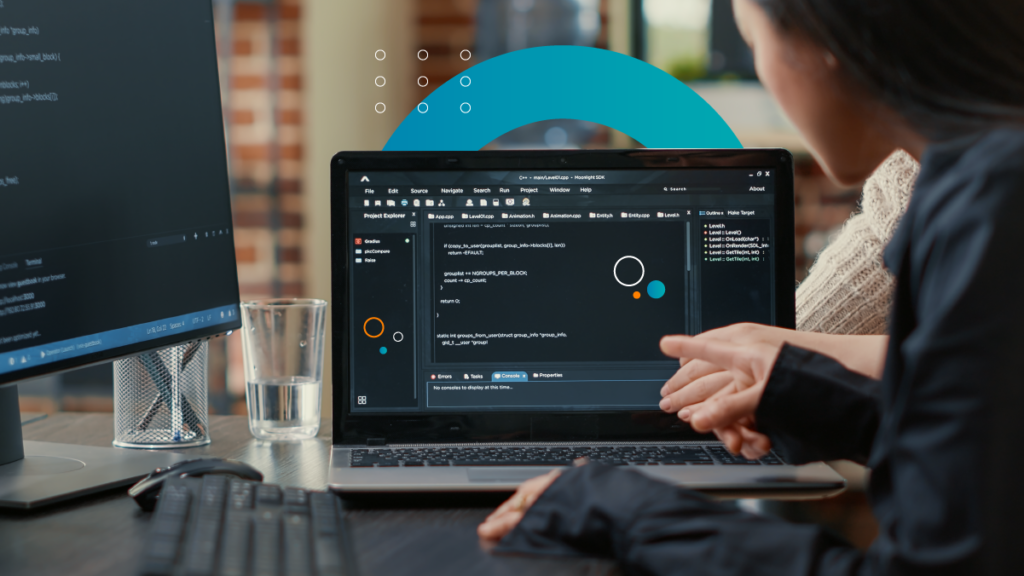
Have you ever wondered how machines can understand and interpret human language? In today’s rapidly evolving technological landscape, artificial intelligence (AI) has become a driving force behind numerous advancements. AI training data services play a pivotal role in equipping AI systems to comprehend and generate human language accurately.
However, despite the growing demand for these services, many still need help to figure out their implications; it’s a complex concept, and we get it. But failing to understand what it’s all about can translate into missed opportunities to capitalise on its benefits.
This article aims to analyse the ins and outs of these solutions and shed light on their capabilities, advantages, and real-world use cases. Ready to dive in? Good.
Understanding AI Training Data Services
If you’re still not an expert on the matter, don’t panic. The following segments will help you to get a more rounded idea. And ultimately, this will help you to recognise which type of solution best suits your needs and can yield the greatest rewards.
The Role of AI Training Data in Enhancing AI Models
In a globalised world, businesses strive to communicate with customers in their preferred languages. That’s why, at the core of AI training data services lies the goal of enhancing the performance of artificial intelligence models, which includes conversational AI and machine learning models.
You wonder why? It’s because these systems rely on vast amounts of annotated data to learn and adapt to human language patterns.
By training AI models on diverse and multilingual datasets, businesses can develop systems that comprehend and generate language with greater accuracy. I.e., AI training data provides the foundation for teaching machines to decipher the intricacies of human language, including syntax, semantics, and context.
#OptimationalTip: Well-trained data retrieved from AI can be a powerful tool for multinational companies as it enables them to overcome language barriers and expand their reach. Learn more about the advances of artificial intelligence in translation.
Are AI Training Data Services the Same as Data Annotation and Data Labelling?
As with many new and technical concepts, it gets tricky to distinguish between them. So, to quickly answer this segment’s key question: No, those three concepts aren’t the same. However, they’re deeply connected.
Here’s a brief explanation of each term.
- AI Training Data Services: It refers to the broad range of solutions to prepare and optimise data for training machine learning models. This can include tasks such as data collection, data preprocessing, data augmentation, and more.
- Data Annotation: It encompasses various methods and techniques used to enrich or enhance data by adding additional information or context. It includes tasks such as data labelling, but can also involve other types of annotations such as bounding, key points, sentiment analysis, and more—find below for more details. Annotation helps to make the data more informative and suitable for training.
- Data Labelling: It’s the process of assigning predefined tags to specific data points or objects within a dataset. It’s about describing certain characteristics or properties of the data. I.e, an image classification task, it’d involve identifying objects with corresponding class labels.
In summary, data labelling is a specific task in data annotation, a broader term encompassing techniques to enhance data. AI training data services prepare and optimise data for AI models, including labelling and annotation.
#OptimationalTip: To be truly valuable, training data requires labelling. And, although the task can be done by automation, oftentimes human-generated labels provide the highest level of accuracy and are frequently the only viable approach.
8 Use Cases and Types of Data Annotation Services
Below is a list of some techniques that make up this service, that, when undertaken by professional linguists, can deliver high-quality output. We made sure to include examples.
- Class Labelling: Assigning predefined tags or categories to data points, typically used for classification tasks. For example, classifying emails as spam.
- Object Labelling: Identifying specific objects within images or videos. For instance, tagging cars, pedestrians, and traffic signs in autonomous driving datasets.
- Semantic Segmentation: Labelling individual pixels or regions within an image to assign them to specific categories. It helps in tasks like identifying different types of vegetation in satellite imagery.
- Sentiment Analysis: Labelling to indicate the sentiment or emotion expressed, such as positive or negative. It’s quite popular in SNS analysis and customer feedback classification.
- Temporal Annotation: Enriching data with information, such as dates, times, or durations. This is crucial for tasks involving time-series analysis, event detection, or historical data processing.
- Audio Annotation: Transcribing and labelling spoken words or segments within audio content. Pretty useful in speech recognition and business transcription.
- Key Point Annotation: Marking specific landmarks within an image or video. For example, tagging facial points for facial recognition.
- Text Annotation: This is about identifying entities, parts of a speech, language, etc. Since it encompasses different AI training data services sub-techniques, we’ll define the most relevant:
- Named Entity Recognition (NER), is about identifying entities, such as names of people, organisations, locations, etc. It enhances information extraction and user intent understanding. For example, tagging named entities in news articles.
- Part-of-Speech (POS) Tagging involves using grammatical tags to help AI grasp the syntactic structure of sentences, improving language understanding and generation. It’s also useful for disambiguating word meanings. I.e., labelling each word in a sentence with its corresponding part of speech, such as noun, verb, adverb, etc.
A Special Case: Natural Language Understanding
If you’re here, you’ve probably heard or read the acronym NLP at least once. Haven’t you?
That’s why we think Natural Language Understanding (NLU) and its relationship with NLP deserves a special room in this conversation.
NLU involves training machine learning models to comprehend and interpret human language by analysing its structure. The goal is to help them extract meaning from text or speech data, including aspects such as grammar, syntax, semantics, context, and intent.
And as you may know, NLU is a fundamental component in various natural language processing (NLP) applications, including chatbots, virtual assistants, language translation, and question-answering systems.
#OptimationalTip: NLU is the sum of several of the techniques mentioned above, with the ultimate goal of enabling the machine to get to grips with the richness of languages.
Top Benefits of AI Data Training Services for International Businesses
As we know, data is essential to make informed decisions and improve our capabilities and overall strategies, no matter what industry for. However, the data we get (disregards of the source) isn’t always clear to interpret, not even for us, humans.
Sadly, data can be messy or incomplete. But the sunny side is that we can give it the order and context it needs, to make sense out of it. With data annotation services, you can aim for that and reap the following benefits:
Increased Efficiency
You can optimise processes and enhance operational efficiency. By training AI models on high-quality annotated data, it’s possible to automate various tasks, streamline workflows, and reduce manual efforts. This translates into cost savings, faster response times, and improved productivity for international operations. I.e., you can leverage an AI chatbot that operates 24/7 and handle large volumes of queries simultaneously.
Enhanced Decision-Making
Another benefit of this type of service is that, combined with data analysis, it enables you to extract valuable insights from multilingual input. For example, sentiment analysis models formed from annotated data help identify customer opinions, assess brand perception and gauge market sentiment. This, in turn, empowers you to develop targeted marketing strategies, optimise client experience and gain a competitive advantage in international markets.
Multilingual Support
By relying on AI training data services, you can train conversational AI systems to make them capable of providing customer support in different languages. In other words, using high-quality data enables you to develop AI systems that understand and respond to customer queries accurately and efficiently, irrespective of the language used.
Improved CX
Collected data enables you to create AI-powered applications that provide personalised recommendations, tailored content and intuitive user interfaces. These apps can anticipate your customers’ needs, provide them with relevant information and improve their overall UX. If you run an international business, this is an invaluable asset for making localisation an integral part of personalisation, which will drive a richer experience.
#OptimationalTip: There are other secondary benefits, such as increasing the quality of the machine translation output. This helps in achieving texts that are better localised to the language variant of your clients and makes the process of professional content editing more time and cost-effective for you.
Head to this article if you want to learn about the perks of AI in the subtitling world.
Wrapping Up: Where There’s Potential, There’s Innovation
As the demand for AI-based solutions continues to rise, understanding their significance is critical for businesses seeking to remain at the forefront of the digital era. By harnessing the power of AI training data services, you can embark on a transformative journey, driving innovation and redefining relationships with customers. These solutions are key in the development of modern technology, enabling machines to learn from examples, just as humans do.
However, challenges arise, such as the need to determine the optimal amount of data, which varies based on several factors, like the desired level of accuracy. For that, partnering with skilled professionals is essential to navigate this landscape, streamline the annotation process, and help you unlock AI’s full potential. Yet, the analysis of the challenges is worth a separate discussion. So, stay tuned for more on that in a future article.
If AI training data sounds like the right fit for you, contact us today. We can guide you with what kind of service you would benefit the most and go for it.